Earphone wearables designed at Cornell convert facial expressions into emoji with nearly 90 per cent accuracy
- C-Face uses earphones connected to a Raspberry Pi and recognises facial expressions to convert them into emoji
- The researchers at Cornell’s SciFi Lab say the technology could help the disabled and others who need hands-free communication
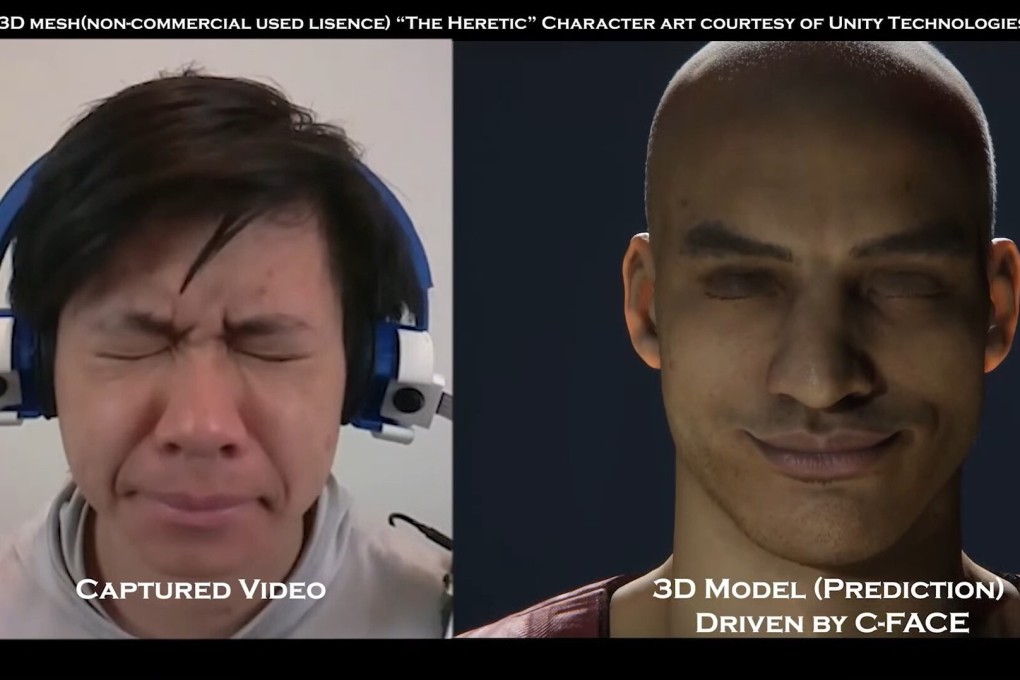
The perfect emoji speaks louder than words, but finding the right one among the more than 3,000 that exist can be difficult. Now a group of researchers discovered a new way to help people find the emoji that best matches their facial expressions, no typing or talking required. And it could have applications beyond just expressive texting.
The invention, created by a team from Cornell University, involves an earphone or headphone system called C-Face. Cameras are attached to each earpiece, recording changes in the contours of the wearer’s face as it moves. The data is processed using deep learning, a branch of AI in which algorithms learn tasks by looking for patterns within a massive amount of data.
Most of the facial changes were found to occur in the mouth, eyes and eyebrows. By following these feature points, researchers said their system was able to reconstruct facial expressions such as a smile, grimace, sneer or frown. It works even for people wearing glasses or medical masks because the contour of the face remains visible.
In the study, participants were also asked to make facial expressions corresponding to some common emoji like “kissy-face” and “sneer”. Based on their faces, the AI-trained system successfully found the right emoji more than 88 per cent of the time.
Researchers said that in the real world, C-Face could allow users to insert an emoji in a message just by imitating it with their face. Since the system tracks the facial expression with the earphones, smartphone users could look away from their screen and continue with other tasks, whether it is jogging or doing the dishes. The small size of the earphones could make them ideal mobile wearables.
“This device is simpler, less obtrusive and more capable than any existing ear-mounted wearable technologies for tracking facial expressions,” said Cheng Zhang, senior author of the research paper and director of Cornell’s SciFi Lab. “In previous wearable technology aiming to recognise facial expressions, most solutions needed to attach sensors on the face.”
Another potential application is silent speech recognition. Participants in the study were asked to mouth several commands to control a music player, such as “play”, “stop” and “next song”. The accuracy was nearly 85 per cent. Researchers suggested that this could be useful not just for the disabled, but also in noisy environments or settings where it is inappropriate to speak.