What every manager should know about artificial intelligence
Already, machine algorithms are predicting how we click, buy, lie, or die. Companies have automated how they mail, call, offer a discount, recommend a product, show an ad, inspect for flaws, and approve a loan.
For Intel, Microsoft, Hewlett-Packard, and the like, Computex in Taipei this week is the Oscars of the information technology world. There is the flurry of product hype, from virtual reality (VR) headsets to the internet of things (IoT) to artificial intelligence (AI) devices. One big topic that everyone has in mind is: machine learning.
The idea of a thinking machine goes back at least as far as 1950, when British computer scientist Alan Turing famously wrote that if a machine was indistinguishable from a human during text-based conversations, then it was “thinking.” Naturally, big tech giants such as Google, Facebook, and IBM, have all set up corporate labs to advance machine learning. At the same time, it’s worth asking; what are the implications for non-tech companies?
If we were to make a list of the most disruptive technologies since the last century, it is likely to include computers, the internet, commercial aviation, automobiles, and container shipping. In each case, the technology creators brought forth new products and services, but other sectors of the economy also benefited from higher productivity. Computers have eased office work, the internet enabled e-commerce, airliners spurred tourism, automobiles encouraged consumers to shop at megastores and containers facilitated global logistics.
Such is the nature of general-purpose technologies. From steam engines to electricity, companies other than technology creators can reap big rewards if they can adapt their business models on time. Sony, Canon, and Nikon do not make microprocessors — that’s Intel’s expertise, But these companies were able to commercialise digital cameras using that technology. Viewed in this light, non-tech companies can also embrace machine learning by making timely, strategic choices. Machine learning isn’t an end in and of itself but a mere enabler, however potent it is. The key is to find creative uses for it.
Already, machine algorithms are predicting how we click, buy, lie, or die. Companies have automated how they mail, call, offer a discount, recommend a product, show an ad, inspect for flaws, and approve a loan. Credit card companies detect in real-time which transactions are likely to be fraudulent. Insurers anticipate which customers are likely to file claims.
The hardest decision among executives is whether the firm should build its machine-learning capability in-house or outsource it to other technology creators. General Electric’s (GE) CEO Jeffrey Immelt calls the dilemma “make or buy.”
“We have lots of good software partners, but, basically, we said we need to do this ourselves. Let’s err on the side of seeing if we could approach it in that way,” he said.
That was when GE began building its digital team in California in 2010.
When pursuing a new capability, like machine learning, it is often difficult, if not impossible, for senior management to articulate a precise market strategy from day one. Experimentation and organisational learning are key. A winning strategy is often recognised only after the fact. Recruit Holdings from Japan was facing exactly that situation.
Recruit Holdings is a classified advertisement, publication and human resources company founded in 1963. As a US$10 billion company (compared to Apple’s some US$200 billion-plus), Recruit cannot afford to become a technology creator.
At the company, therefore, senior executives focus on creating an environment to facilitate the exchange of ideas across business units. Besides setting up a mini machine-learning lab, the company dispatches most of its data analysts to business units so they become the conduit to the laboratory. Business strategy-making becomes a bottom-up exercise rather than being imposed from top-down.
In a world where opportunities for innovation are abundant, executives must be willing to cultivate a critical mass of future thinkers who are steeped in machine learning, place them close to businesses, and charter them to do something bold, lest they be left behind. No one can afford to be unprepared.
Howard Yu is professor of strategic management and innovation at IMD.
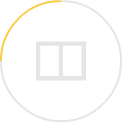