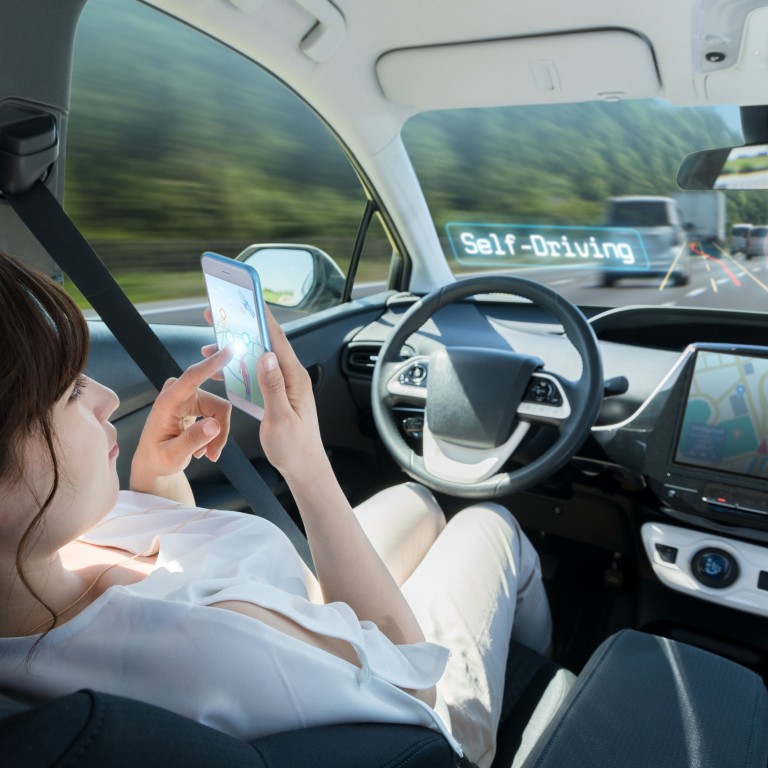
Chinese researchers just won a top AI award. Will their algorithm be driving your next car?
- In tests, an autonomous driving technology based on a large-scale AI model outperformed similar systems, like Tesla’s Full Self-Driving
- The research could steer the tech for self-driving vehicles in an entirely new direction, expert says
Not long ago, the idea of vehicles that could drive themselves seemed like a dreamy, futuristic vision of transport. Then suddenly, vehicles with assisted driving were on the road. But the race to make fully self-driving vehicles, powered by autonomous driving systems, truly workable – and trustworthy – is far from the finish line.
Their work, which could point to an entirely new direction for the industry, recently won a best paper award at a top academic conference.
According to some experts, the technology has the potential to significantly outperform systems currently being tested in vehicles, including Tesla’s Full Self-Driving (FSD).
At the Conference on Computer Vision and Pattern Recognition (CVPR) held by the Institute of Electrical and Electronics Engineers (IEEE) in Vancouver on June 21, a joint project by researchers from the Shanghai AI Lab, Wuhan University and SenseTime was awarded the event’s prize for best paper.
This year’s CVPR – a top annual event in the field of artificial intelligence and computer perception – received 9,155 submissions. Only a quarter of them were accepted, and just two of the submissions were worthy of the best paper award.
Faced with formidable competition from research submitted by leading universities and tech giants, including Google, Stanford and Cornell, it was the first time that Chinese scientists won the award.
Central to the research was a new autonomous driving algorithm called Unified Autonomous Driving (UniAD), a design that, according to testing, outperformed other mainstream autonomous driving models, including Tesla’s FSD.
In simulated driving tests using street scene data collected from Boston and Singapore, UniAD outperformed other autonomous systems by 20 to 30 per cent across various parameters, including tracking and prediction of other objects.
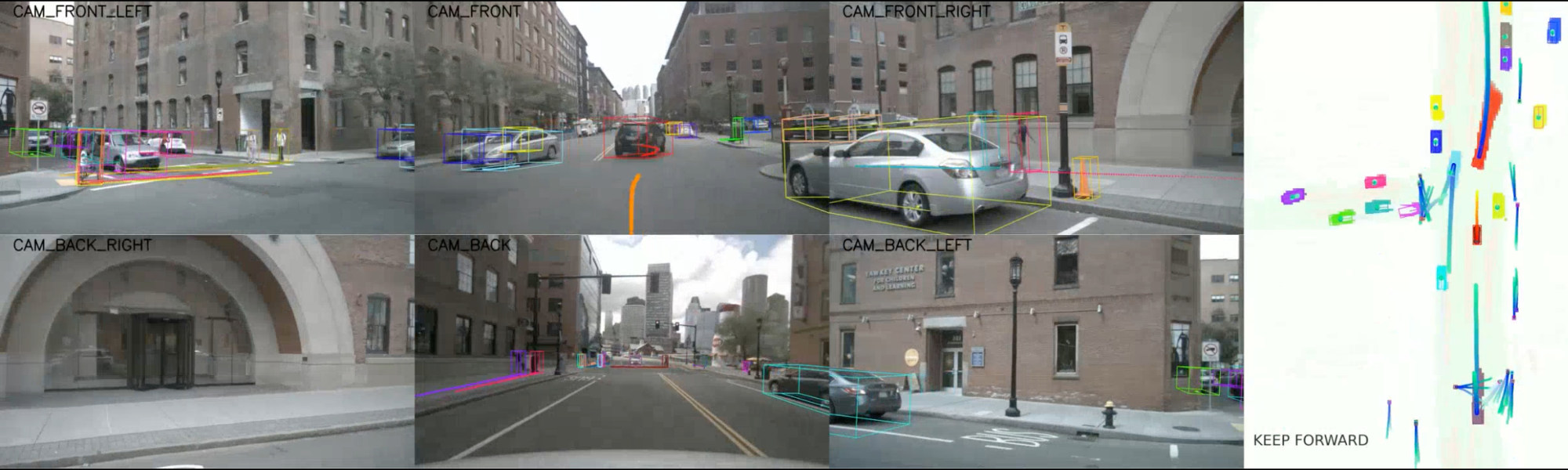
What sets UniAD apart from most other industry solutions is that – for the first time – it integrates perception and decision-making, resulting in a driving system that follows a planning-oriented philosophy.
“To the best of our knowledge, UniAD is the first work to comprehensively investigate the joint cooperation of such a variety of tasks including perception, prediction and planning in the field of autonomous driving,” Li Hongyang, lead scientist with Shanghai AI Lab, said in the paper.
Modern autonomous driving systems integrate aspects of both the car industry and artificial intelligence, which include a series of tasks such as detection, tracking and mapping. Existing autonomous driving algorithms can be broadly divided into three categories.
The most common modular designs complete perception, prediction and planning tasks separately. While the development of each module is more flexible, such designs risk the loss of information across modules.
Some AI scientists say the large-scale AI model could be the ultimate solution for autonomous driving. Since it is based on a more direct decision chain, the system could drastically reduce the chances of information errors, resulting in higher performance potentials.
UniAD’s algorithm performance was based on real-world scenario data sets from nuScenes, a large public data set collected on actual roads. It has served as a benchmark for many perception algorithms and autonomous driving systems.
According to the paper, UniAD received top marks in all tests using nuScenes. For instance, multi-object tracking accuracy was 20 per cent better than a previous best, and the error rate in motion forecasting and planning was reduced by 38 and 28 per cent, respectively.
While the promising results signal an advance in autonomous driving technology, the nuScenes data set is relatively small compared to real-world self-driving scenarios. That opens the possibility that there could be a considerable gap between UniAD’s test performance and its practical application.
The FSD system has seen numerous iterations in simulation training. As of May, the total distance driven for Tesla’s global fleet had exceeded 100 billion miles (161 billion km), and the driver behaviour data voluntarily provided continues to help refine the FSD algorithm.
But, according to its developers, the UniAD model’s more comprehensive design gives it the potential to become a next-generation autonomous driving technology. “Because of its full interpretability, safety, and continuous iteration across multiple modules, UniAD is the most promising end-to-end model for practical deployment,” Shanghai AI Lab’s Li said.
As the field of autonomous driving rapidly evolves, it remains highly competitive, with various companies racing to develop systems that they hope will be a game changer. The researchers behind the UniAD system say their technology deserves further exploration.
“We hope this work can shed some light on the target-driven design for an autonomous driving system, and provide a starting point for coordinating the many driving tasks,” Li said in the paper.
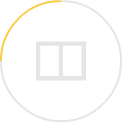